Causal relationship between gut microbiota and pancreatic cancer: a two-sample Mendelian randomisation study
Highlight box
Key findings
• Specific gut microbiota with a potential causal relationship with the occurrence and development of pancreatic cancer were identified at the gene prediction level.
What is known and what is new?
• Observational epidemiology studies suggested a relationship between the gut microbiome and pancreatic cancer. However, the causal relationship remains unclear because of confounding factors and reverse causality.
• This study revealed the causal relationship between the gut microbiome and pancreatic cancer by Mendelian randomization.
What is the implication, and what should change now?
• This study provides a theoretical basis for the aetiology of pancreatic cancer and contributes to the prevention and treatment of pancreatic cancer.
Introduction
Pancreatic cancer is a common malignancy of the digestive system. Due to its concealed incidence, rapid metastasis of cancer cells, poor treatment effects, and poor prognosis, the 5-year survival rate is only 10% (1). In recent years, the morbidity and mortality of pancreatic cancer have substantially increased, and pancreatic cancer is expected to become the second leading cause of cancer-related deaths in the United States by 2030 (2). Therefore, there is an urgent need to identify its causes and implement preventive measures. Chronic pancreatitis, smoking, drinking and family genetic history are risk factors for pancreatic cancer (3-5). The continuous discussion of the pathogenesis of pancreatic cancer led to an increasing number of studies that suggested an imbalance in gut microbiota homeostasis also leads to the pathogenesis of pancreatic cancer.
The gut microbiota is the largest microecosystem in the human body and is closely related to human health and disease (6). The gut microbiota maintains various physiological functions and plays a positive role in the host immune system by maintaining immune homeostasis. Once the gut microbiota is in a state of metabolic disorder, the imbalance and functional regulation of intestinal bacteria leads to a variety of diseases (7), such as obesity, diabetes, immune diseases, cardiovascular diseases, and nervous system diseases (8-12). In recent years, several studies have revealed that the gut microbiota is closely related to many malignant tumours (13,14). Intestinal flora imbalance can activate the immune system, promote the proliferation of inflammatory cells, inhibit apoptosis, and induce DNA chain damage, oxidative stress, and tumorigenesis. Although some observational studies have revealed a relationship between the gut microbiota and pancreatic cancer, it is difficult to confirm a causal relationship between the gut microbiota and pancreatic cancer through observational studies due to potential confounding factors and reverse causality.
In 1986, Katan proposed the Mendelian randomisation (MR) (15). It is used to estimate the causal relationship between exposure and outcomes by means of gene variations, such as single nucleotide polymorphisms (SNPs), as instrumental variables (IVs) for exposure factors. During human gametogenesis, specific SNP alleles are randomly assigned to ooplasm/sperm cells; therefore, genetic variation is not related to potential mixed environmental exposures. Additionally, genetic variation is not affected by the disease throughout its life cycle, which avoids the possibility of a reverse causal association. In this study, a two-sample MR method was used to study the role of gut microbiota in the occurrence and development of pancreatic cancer. The purpose of this study was to explore the potential causal relationship between gut microbiota and pancreatic cancer and to provide a theoretical basis for the aetiology of pancreatic cancer to prevent its occurrence. We present this article in accordance with the STROBE reporting checklist (available at https://apc.amegroups.com/article/view/10.21037/apc-23-15/rc).
Methods
MR
A two-sample MR method was used to extract SNPs related to the gut microbiota as IVs to explore the causal relationship between gut microbiota and pancreatic cancer. The selected IVs needed to meet the following three key assumptions (Figure 1): (I) relevance assumption: IVs must have a strong correlation with exposure. (II) Independence assumption: IVs are unrelated to confounding factors and those related to confounding factors have to be removed. (III) Exclusivity assumption: IVs can only affect the outcome through exposure but cannot be directly related to the outcome (16).
Data source of exposure and outcome
The complete genome-wide association studies (GWAS) summary of the gut microbiota was based on the MiBioGen consortium meta-analysis of 18,340 people in 24 queues, most of whom were of European origin (n=13,266). A total of 211 gut microbiota and 122,110 related SNPs were recorded (17). The European population was selected and analysed using the Open GWAS database (https://gwas.mrcieu.ac.uk/). The GWAS number for pancreatic cancer was derived from the latest Finn Gen Union R9 Distribution data (18) (https://www.finngen.fi/en), which is also based on the European population, including 287,829 subjects; 692 patients with pancreatic cancer and 287,137 healthy controls.
The selection of IVs
The IVs for analysis must be closely related to the exposure factors. There were few results in which the SNP of each level of the gut microbiota reached the genome-wide statistical significance threshold (P<5×10−8), therefore, to ensure the full screening of IVs, the SNP with a P value less than the whole locus significance level (P<1×10−5) was selected. F-statistics were used to evaluate the strength of IVs (19,20). F-value formula: F = (beta/se)2 (21). IVs with an F-value of less than 10 were excluded to ensure the intensity of the association between the IV and exposure. The selected IVs needed to satisfy the independence test, and to avoid the influence of linkage disequilibrium in SNP on the analysis results, the PLINK clustering method was used to set the linkage disequilibrium parameter (R2) of SNP to 0.001 and the genetic distance to 10,000 kB (22). In addition, to only include SNPs that are relevant to the outcome through exposure, we passed the screened phenotypes that were significantly associated with SNPs according to the library through PhenoScanner (V2) (https://ldlink.nci.nih.gov/?tab=ldtrait) and determined whether these phenotypes were confounding factors for pancreatic cancer based on previously published studies. Glycosuria, chronic pancreatitis, smoking, alcohol consumption, and familial genetic history are risk factors for pancreatic adenocarcinoma (4,5). The SNPs associated with these risk factors were excluded. Finally, to avoid distortion of chain direction or allele coding, SNPs with a palindromic structure were automatically excluded during the analysis, and ambiguous and duplicated SNPs were deleted during the coordination process. The selection of these IVs ensures the reliability of the results.
MR analysis
This study used various two-sample MR methods, including inverse variance weighted (IVW), MR-Egger, weighted median, and weighted modes. IVW is considered the standard method for collecting data in MR (23,24). This method uses the Wald ratio to estimate the progressive causal effect of each included tool SNP and then performs a weighted summary analysis (25). The MR-Egger method is used based on the hypothesis that the multiple effects of IVs on exposure factors are independent of each other. The method can be used to test causality and estimate causal effects. However, the statistical efficiency of the causal estimation of the MR-Egger method is lower than that of the IVW mode, and the confidence interval is wider. If the above assumptions are not satisfied, the weighted mode has greater ability to detect causal effects, with a smaller deviation and lower class I error rate than the MR-Egger method. However, this method provides statistically weaker results than the IVW and weighted median modes. The weighted median mode, which uses the median estimated by the IVW ratio, is more robust for outliers than the IVW mode and the MR-Egger method, and requires that at least 50% of the weights contributed by genetic variation are valid for statistical calculation (26). If the results of these methods are inconsistent, priority should be given to IVW as the primary result. However, it is necessary for the beta values of the results from these methods to follow the same direction.
The intercept terms of the MR-Egger regression and MR-PRESSO tests were used to test the horizontal multiplicity and outliers of SNP. If P>0.05, it indicates that significant horizontal pleiotropy does not exist. Compared with MR-Egger, MR-PRESSO has a higher precision. It not only calculates the horizontal range of IVs, but can also calculate the effect between exposure and outcome after removing outliers and test the results before and after correction (27). Cochran’s Q statistics were calculated using IVW and MR-Egger regressions. No significant heterogeneity was indicated by P>0.05. The leave-one-out method was used to eliminate the SNPs individually to observe whether they had an impact on the analysis results, and draw a forest map (25). All statistical analyses were performed using R (version 4.3.0) through the two-sample MR package (28) and MRPRESSO (27). A flowchart of the study is shown in Figure 2.
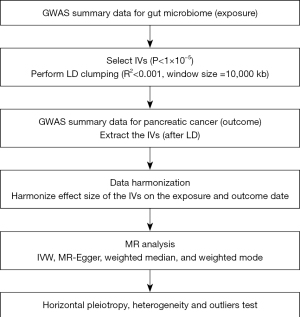
The study was conducted in accordance with the Declaration of Helsinki (as revised in 2013). No additional ethics approval or informed consent was required due to our study was based on public databases.
Results
The selection of IVs
After the PLINK clustering process of unbalanced interlocking, the linkage imbalance effect of specific flora was eliminated, and a series of quality controls, such as weakly related variables (F <10) of exposure factors were removed, 2,559 SNPs were identified as IVs (tables available at https://cdn.amegroups.cn/static/public/apc-23-15-1-5.zip). These SNPs were divided into phylum, class, family, order, and genus according to five levels, with 125, 224, 280, 434, and 1,496 SNPs respectively. To ensure that the effects of SNPs on exposure correspond to the same alleles as those obtained via the results, and to avoid distortions in chain direction or allele coding, we deleted palindromic SNPs (for example, alleles with A/G/T or G/C) through the code. We then compared the alleles with the human genome reference sequence (construction 37) during the coordination process and deleted ambiguous and duplicated SNPs. Finally, a new set of data that integrates the relevant SNPs of exposure and outcome was obtained (tables available at https://cdn.amegroups.cn/static/public/apc-23-15-6-10.zip). Using the PhenoScanner (V2) database, rs11979110 was related to diabetes, rs79535861 was related to self-reported malignant melanoma, and rs4680035 was related to lymphoid malignancies, and the confounding factors were removed and re-analysed. Palindromic SNPs were removed simultaneously.
Two-sample MR analysis
IVW assessment showed a causal relationship between nine types of intestinal bacteria and pancreatic cancer (Figure 3). Among them, the Erysipelotrichia class [odds ratio (OR) =2.33; 95% confidence interval (CI): 1.26–4.30; P=0.007], Erysipelotrichaceae family (OR =2.33; 95% CI: 1.26–4.30; P=0.007), Veillonellaceae family (OR =1.53; 95% CI: 1.01–2.31; P=0.05), Alloprevotella genus (OR =1.64; 95% CI: 1.05–2.54; P=0.03), and Odoribacter genus (OR =2.46; 95% CI: 1.15–5.25; P=0.02). The Parabacteroides genus (OR =3.18; 95% CI: 1.32–7.66; P=0.01), and Erysipelotrichales order (OR =2.33; 95% CI: 1.26–4.30; P=0.007) were associated with increased risk of pancreatic cancer. The Bacteroidales family S24-7 group (OR =0.61; 95% CI: 0.38–0.98; P=0.04) and Lactococcus genus (OR =0.63; 95% CI: 0.41–0.98; P=0.04) were associated with reduced risk of pancreatic cancer. Additionally, three methods, MR-Egger, weighted median, and weighted mode, were used to assess the causal impact of the gut microbiota on pancreatic cancer. The results were similar to the IVW results (Table 1).
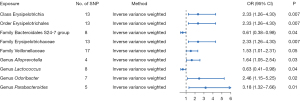
Table 1
Exposure | SNP, n | Methods | Beta | Se | OR (95% CI) | P |
---|---|---|---|---|---|---|
Class Erysipelotrichia | 13 | IVW | 0.85 | 0.31 | 2.33 (1.26–4.30) | 0.007 |
13 | MR-Egger | 2.27 | 1.35 | 9.67 (0.69–135.24) | 0.12 | |
13 | Weighted median | 0.72 | 0.43 | 2.06 (0.88–4.82) | 0.10 | |
13 | Weighted mode | 0.84 | 0.71 | 2.32 (0.57–9.39) | 0.26 | |
Order Erysipelotrichales | 13 | IVW | 0.85 | 0.31 | 2.33 (1.26–4.30) | 0.007 |
13 | MR-Egger | 2.27 | 1.35 | 9.67 (0.69–135.24) | 0.12 | |
13 | Weighted median | 0.72 | 0.43 | 2.06 (0.89–4.77) | 0.09 | |
13 | Weighted mode | 0.84 | 0.67 | 2.32 (0.62–8.65) | 0.23 | |
Family Bacteroidales S24-7 group | 8 | IVW | −0.49 | 0.24 | 0.61 (0.38–0.98) | 0.04 |
8 | MR-Egger | −0.29 | 0.98 | 0.75 (0.11–5.07) | 0.78 | |
8 | Weighted median | −0.38 | 0.30 | 0.68 (0.38–1.24) | 0.21 | |
8 | Weighted mode | −0.32 | 0.45 | 0.72 (0.30–1.74) | 0.49 | |
Family Erysipelotrichaceae | 13 | IVW | 0.85 | 0.31 | 2.33 (1.26–4.30) | 0.007 |
13 | MR-Egger | 2.27 | 1.35 | 9.67 (0.69–135.24) | 0.12 | |
13 | Weighted median | 0.72 | 0.43 | 2.06 (0.89–4.74) | 0.09 | |
13 | Weighted mode | 0.84 | 0.71 | 2.32 (0.58–9.35) | 0.26 | |
Family Veillonellaceae | 17 | IVW | 0.43 | 0.22 | 1.53 (1.01–2.31) | 0.05 |
17 | MR-Egger | 0.66 | 0.43 | 1.54 (1.00–2.35) | 0.05 | |
17 | Weighted median | 0.42 | 0.31 | 1.93 (0.83–4.47) | 0.15 | |
17 | Weighted mode | 0.66 | 0.39 | 1.52 (0.83–2.79) | 0.18 | |
Genus Alloprevotella | 4 | IVW | 0.49 | 0.22 | 1.64 (1.05–2.54) | 0.03 |
4 | MR-Egger | 0.85 | 2.30 | 2.35 (0.03–213.28) | 0.75 | |
4 | Weighted median | 0.47 | 0.27 | 1.60 (0.95–2.70) | 0.08 | |
4 | Weighted mode | 0.34 | 0.38 | 1.40 (0.67–2.93) | 0.44 | |
Genus Lactococcus | 8 | IVW | −0.46 | 0.22 | 0.63 (0.41–0.98) | 0.04 |
8 | MR-Egger | −0.01 | 1.09 | 0.99 (0.12–8.39) | >0.99 | |
8 | Weighted median | −0.43 | 0.26 | 0.65 (0.39–1.08) | 0.09 | |
8 | Weighted mode | −0.42 | 0.37 | 0.66 (0.32–1.36) | 0.29 | |
Genus Odoribacter | 7 | IVW | 0.90 | 0.39 | 2.46 (1.15–5.25) | 0.02 |
7 | MR-Egger | 1.84 | 1.26 | 6.27 (0.53–73.54) | 0.20 | |
7 | Weighted median | 0.71 | 0.51 | 2.04 (0.75–5.52) | 0.16 | |
7 | Weighted mode | 0.59 | 0.72 | 1.81 (0.44–7.46) | 0.45 | |
Genus Parabacteroides | 5 | IVW | 1.16 | 0.45 | 3.18 (1.32–7.66) | 0.01 |
5 | MR-Egger | 4.51 | 2.80 | 91.19 (0.38–21,991.68) | 0.21 | |
5 | Weighted median | 1.49 | 0.57 | 4.43 (1.45–13.49) | 0.009 | |
5 | Weighted mode | 1.62 | 0.74 | 5.05 (1.18–21.61) | 0.10 |
MR, Mendelian randomisation; SNP, single nucleotide polymorphism; Se, standard error; OR, odds ratio; CI, confidence interval; IVW, inverse variance weighted.
The results of the MR-Egger intercept and MR-PRESSO global tests showed that there was no horizontal pleiotropy in the IV of the nine gut microbiota groups associated with pancreatic cancer (pMR-Egger intercept >0.05 and global pMR-PRESSO >0.05). Additionally, the results of Cochran’s Q test showed no significant heterogeneity in the selected SNP (P>0.05) (Table 2). Furthermore, the scatter plot (Figure 4) revealed the effect of gut microbiota on pancreatic cancer. The leave-one-out methods (Figure 5) showed that in addition to Erysipelotrichia, Erysipelotrichaceae, and Erysipelotrichales, some single SNPs may have dominated the positive results.
Table 2
Exposure | Horizontal pleiotropy | Heterogeneity | ||||||||||||
---|---|---|---|---|---|---|---|---|---|---|---|---|---|---|
MR PRESSO | MR-Egger | IVW | MR-Egger | |||||||||||
RSSobs | P value | Egger_intercept | Se | P value | Q | Q_df | Q_pval | Q | Q_df | Q_pval | ||||
Class Erysipelotrichia | 14.458 | 0.44 | −0.089 | 0.082 | 0.30 | 12.099 | 12 | 0.44 | 10.921 | 11 | 0.45 | |||
Order Erysipelotrichales | 14.458 | 0.43 | −0.089 | 0.082 | 0.30 | 12.099 | 12 | 0.44 | 10.921 | 11 | 0.45 | |||
Family Bacteroidales S24-7 group | 2.798 | 0.95 | −0.021 | 0.096 | 0.84 | 2.161 | 7 | 0.95 | 2.115 | 6 | 0.91 | |||
Family Erysipelotrichaceae | 14.458 | 0.44 | −0.089 | 0.082 | 0.30 | 12.099 | 12 | 0.44 | 10.921 | 11 | 0.45 | |||
Family Veillonellaceae | 16.014 | 0.60 | −0.021 | 0.034 | 0.55 | 14.193 | 16 | 0.58 | 13.822 | 15 | 0.54 | |||
Genus Alloprevotella | 1.480 | 0.86 | −0.053 | 0.331 | 0.89 | 0.814 | 3 | 0.85 | 0.789 | 2 | 0.67 | |||
Genus Lactococcus | 12.132 | 0.26 | −0.063 | 0.150 | 0.69 | 9.843 | 7 | 0.20 | 9.560 | 6 | 0.14 | |||
Genus Odoribacter | 9.387 | 0.38 | −0.073 | 0.093 | 0.47 | 6.923 | 6 | 0.33 | 6.162 | 5 | 0.29 | |||
Genus Parabacteroides | 3.156 | 0.76 | −0.295 | 0.242 | 0.31 | 2.059 | 4 | 0.73 | 0.582 | 3 | 0.90 |
MR, Mendelian randomisation; IVW, inverse variance weighted; RSSobs, MR-PRESSO globe test; MR-PRESSO, MR pleiotropy residual sum and outlier; Se, standard error; df, degree of freedom.
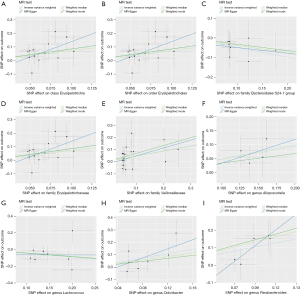
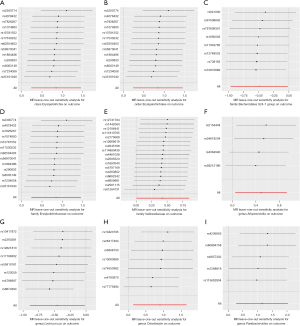
Discussion
Based on a new large GWAS database, to our knowledge, this study used the MR method for the first time to explore the causal relationship between gut microbiota and pancreatic cancer. Seven types of gut microbiota were associated with an increased risk of pancreatic cancer, and two types were associated with a lower risk of pancreatic cancer.
The pancreas is generally considered a sterile organ, which contains many proteases and is highly alkaline; most microorganisms cannot survive in the pancreatic juice. However, using a 16SrRNA fluorescence probe and real-time quantitative polymerase chain reaction (qPCR), it was found that the number of bacteria in the pancreas of patients with pancreatic cancer increased 1,000 times compared to that in normal pancreatic tissue (29). Pushalkar et al. (30) analysed the bacterial composition of faecal samples from 63 individuals, including 32 patients with pancreatic cancer and 31 healthy controls. The top three bacteria in the faeces of patients with pancreatic cancer were Proteus species, synergistic flora, and archaea, whereas the faeces of the healthy control group were mainly composed of Bacteroides, Clostridium, and actinomycetes. Additionally, Half et al. (31) had a considerable decrease in the number of bacteria belonging to the Pleurobacterium genus in the faeces of patients with pancreatic cancer. These findings suggest that the gut microbiota of patients with pancreatic cancer substantially differs from that of healthy controls. The ecological imbalance of the gut microbiota, interference of normal metabolic function, and toxic metabolites are closely related to the occurrence of pancreatic cancer and even affect the prognosis (32). However, these studies mainly collected faeces from patients with pancreatic cancer, and the results obtained by histological analysis were based on cross-sectional studies. Differences in faecal or rectal bacterial abundance and composition between pancreatic cancer patients and healthy controls could not explain the causal relationship between the two. This MR study found that Erysipelotrichia, Erysipelotrichaceae, Veillonellaceae, Alloprevotella, Odoribacter, Parabacteroides, and Erysipelotrichales were associated with an increased risk of pancreatic cancer. The Bacteroidales S24-7 group and Lactococcus were associated with a lower risk of pancreatic cancer. The results were consistent with those of the IVW, weighted median method, and MR-Egger regression. The data used in this study came from two large-scale GWAS studies, and the included IVs were screened using the PhenoScanner database; their F statistics were all greater than 10, and there was no obvious heterogeneity or multiple effects among IVs. These conditions ensured the robustness of the MR results.
This study confirmed the role of Bacteroides in reducing the risk of pancreatic cancer because Bacteroides can produce large amounts of butyrate, which can achieve anti-tumor effects by inhibiting histone deacetylase and telomerase activity. In addition, butyrate can induce regulatory T-cell proliferation, produce anti-inflammatory cytokines, reduce DNA oxidative damage, induce apoptosis of DNA-damaged cells, and prevent tumor cell growth. Regulating the level of butyric acid by interfering with the intestinal flora may be helpful in the prevention and treatment of pancreatic cancer. However, some of the results of this study differ from those of previous studies, probably because most of the previous studies were case-control studies, in which the time and results of exposure are difficult to confirm. In addition, in observational studies, the association between the intestinal flora and pancreatic cancer is easily affected by confounding factors such as age, environment, dietary patterns, and lifestyle, which are difficult to control. These conditions limit causal inferences with respect to the intestinal flora and pancreatic cancer.
However, the complex mechanism between gut microbiota and pancreatic cancer requires further exploration. In recent years, some studies have shown that gut microbiota imbalance produces related inflammatory mediators, which activate the body’s immune function and related signalling pathways, induce DNA chain damage and oxidative stress response (33,34), thus further inducing tumourigenesis. Therefore, the intervention of gut microbiota in the occurrence of pancreatic tumours is not a direct effect of gut microbiota on the pancreas, but an indirect intervention through inflammation and immune response (35,36). However, injured acinar cells promote inflammatory response and produce inflammatory mediators by releasing digestive enzymes and inflammatory mediators, and cytokines activate inflammatory cytokines TNF-α and IL-1β produced by macrophages, which further aggravate cell injury and induce tumour cells to produce (37). TNF-α also promotes PD-L1 expression in pancreatic cancer cells mediated by NF-κB. This leads to poor prognosis for pancreatic cancer (38). Overall, the immune system maintains a steady state with the gut microbiota, and an imbalance in the gut microbiota activates the immune system, promotes the proliferation of inflammatory cells, and inhibits apoptosis. As our research was mainly based on correlation analysis, we cannot prove the underlying mechanisms by which bacteria affect pancreatic cancer, and these mechanisms need to be further studied.
This study has limitations as follows: (I) the database included in this study is based on a European population, which may produce demographic bias, and the results may not be applicable to other races or populations without further stratification analysis based on sex, age, menopausal status, and other factors; (II) a small number of IVs that met the strict threshold (P<5×10−8), therefore, a relatively loose threshold (P<1×10−5) was used to filter the IVs; (III) the number of pancreatic cancer cases was relatively small; therefore, it is necessary to analyse GWAS summary data based on a larger sample size in the future to increase the credibility of the results; and (IV) only the causal relationship between exposure factors and the final disease was inferred, and no basic research was conducted to explore its mechanism or pathophysiological basis.
Conclusions
Overall, nine potential causal relationships were identified by the MR analysis in 211 gut microbiota and pancreatic cancer. Erysipelotrichi, Erysipelotrichaceae, Veillonellaceae, Alloprevotella, Odoribacter, Parabacteroides, and Erysipelotrichales are associated with an increased risk of pancreatic cancer. The Bacteroidales S24-7 group and Lactococcus are associated with a reduced risk of pancreatic cancer. The causal relationship between some exposure factors and pancreatic cancer remains controversial due to some inconsistent results with previous studies compared with this study. Therefore, further studies with larger sample sizes, boarder population demographics, pathophysiological factors are required. Nevertheless, this study will have an important, positive impact on the prevention and treatment of pancreatic cancer, not only in public health, but also in clinical practice.
Acknowledgments
We want to acknowledge all the participants and investigators for contributing and sharing summary-level data on GWAS. We also want to acknowledge the participants and investigators of the FinnGen study.
Funding: This work was supported by
Footnote
Reporting Checklist: The authors have completed the STROBE reporting checklist. Available at https://apc.amegroups.com/article/view/10.21037/apc-23-15/rc
Data Sharing Statement: Available at https://apc.amegroups.com/article/view/10.21037/apc-23-15/dss
Peer Review File: Available at https://apc.amegroups.com/article/view/10.21037/apc-23-15/prf
Conflicts of Interest: Both authors have completed the ICMJE uniform disclosure form (available at https://apc.amegroups.com/article/view/10.21037/apc-23-15/coif). The authors have no conflicts of interest to declare.
Ethical Statement: The authors are accountable for all aspects of the work in ensuring that questions related to the accuracy or integrity of any part of the work are appropriately investigated and resolved. The study was conducted in accordance with the Declaration of Helsinki (as revised in 2013). No additional ethics approval or informed consent was required due to our study was based on public databases.
Open Access Statement: This is an Open Access article distributed in accordance with the Creative Commons Attribution-NonCommercial-NoDerivs 4.0 International License (CC BY-NC-ND 4.0), which permits the non-commercial replication and distribution of the article with the strict proviso that no changes or edits are made and the original work is properly cited (including links to both the formal publication through the relevant DOI and the license). See: https://creativecommons.org/licenses/by-nc-nd/4.0/.
References
- Siegel RL, Miller KD, Fuchs HE, et al. Cancer Statistics, 2021. CA Cancer J Clin 2021;71:7-33. Erratum in: CA Cancer J Clin 2021;71:359. [Crossref] [PubMed]
- Rahib L, Smith BD, Aizenberg R, et al. Projecting cancer incidence and deaths to 2030: the unexpected burden of thyroid, liver, and pancreas cancers in the United States. Cancer Res 2014;74:2913-21. [Crossref] [PubMed]
- Thomas RM, Jobin C. Microbiota in pancreatic health and disease: the next frontier in microbiome research. Nat Rev Gastroenterol Hepatol 2020;17:53-64. [Crossref] [PubMed]
- Liu C, Zhang J, Li M, et al. Alterations and structural resilience of the gut microbiota under dietary fat perturbations. J Nutr Biochem 2018;61:91-100. [Crossref] [PubMed]
- Yadav D, Lowenfels AB. The epidemiology of pancreatitis and pancreatic cancer. Gastroenterology 2013;144:1252-61. [Crossref] [PubMed]
- Heintz-Buschart A, Wilmes P. Human Gut Microbiome: Function Matters. Trends Microbiol 2018;26:563-74. [Crossref] [PubMed]
- Fan Y, Pedersen O. Gut microbiota in human metabolic health and disease. Nat Rev Microbiol 2021;19:55-71. [Crossref] [PubMed]
- Maruvada P, Leone V, Kaplan LM, et al. The Human Microbiome and Obesity: Moving beyond Associations. Cell Host Microbe 2017;22:589-99. [Crossref] [PubMed]
- Wu H, Tremaroli V, Schmidt C, et al. The Gut Microbiota in Prediabetes and Diabetes: A Population-Based Cross-Sectional Study. Cell Metab 2020;32:379-390.e3. [Crossref] [PubMed]
- Fung TC, Olson CA, Hsiao EY. Interactions between the microbiota, immune and nervous systems in health and disease. Nat Neurosci 2017;20:145-55. [Crossref] [PubMed]
- Tang WH, Kitai T, Hazen SL. Gut Microbiota in Cardiovascular Health and Disease. Circ Res 2017;120:1183-96. [Crossref] [PubMed]
- Sorboni SG, Moghaddam HS, Jafarzadeh-Esfehani R, et al. A Comprehensive Review on the Role of the Gut Microbiome in Human Neurological Disorders. Clin Microbiol Rev 2022;35:e0033820. [Crossref] [PubMed]
- Liu M, Xie W, Wan X, et al. Clostridium butyricum modulates gut microbiota and reduces colitis associated colon cancer in mice. Int Immunopharmacol 2020;88:106862. [Crossref] [PubMed]
- Akshintala VS, Talukdar R, Singh VK, et al. The Gut Microbiome in Pancreatic Disease. Clin Gastroenterol Hepatol 2019;17:290-5. [Crossref] [PubMed]
- Katan MB. Apolipoprotein E isoforms, serum cholesterol, and cancer. 1986. Int J Epidemiol 2004;33:9. [Crossref] [PubMed]
- Burgess S, Small DS, Thompson SG. A review of instrumental variable estimators for Mendelian randomization. Stat Methods Med Res 2017;26:2333-55. [Crossref] [PubMed]
- Kurilshikov A, Medina-Gomez C, Bacigalupe R, et al. Large-scale association analyses identify host factors influencing human gut microbiome composition. Nat Genet 2021;53:156-65. [Crossref] [PubMed]
- Kurki MI, Karjalainen J, Palta P, et al. FinnGen provides genetic insights from a well-phenotyped isolated population. Nature 2023;613:508-18. [Crossref] [PubMed]
- Burgess S, Thompson SGCRP CHD Genetics Collaboration. Avoiding bias from weak instruments in Mendelian randomization studies. Int J Epidemiol 2011;40:755-64. [Crossref] [PubMed]
- Sanderson E, Spiller W, Bowden J. Testing and correcting for weak and pleiotropic instruments in two-sample multivariable Mendelian randomization. Stat Med 2021;40:5434-52. [Crossref] [PubMed]
- Huang W, Xiao J, Ji J, et al. Association of lipid-lowering drugs with COVID-19 outcomes from a Mendelian randomization study. Elife 2021;10:e73873. [Crossref] [PubMed]
- Meng L, Wang Z, Ming YC, et al. Are micronutrient levels and supplements causally associated with the risk of Alzheimer's disease? A two-sample Mendelian randomization analysis. Food Funct 2022;13:6665-73. [Crossref] [PubMed]
- Bowden J, Davey Smith G, Haycock PC, et al. Consistent Estimation in Mendelian Randomization with Some Invalid Instruments Using a Weighted Median Estimator. Genet Epidemiol 2016;40:304-14. [Crossref] [PubMed]
- Lin Z, Deng Y, Pan W. Combining the strengths of inverse-variance weighting and Egger regression in Mendelian randomization using a mixture of regressions model. PLoS Genet 2021;17:e1009922. [Crossref] [PubMed]
- Burgess S, Butterworth A, Thompson SG. Mendelian randomization analysis with multiple genetic variants using summarized data. Genet Epidemiol 2013;37:658-65. [Crossref] [PubMed]
- Walker VM, Davies NM, Hemani G, et al. Using the MR-Base platform to investigate risk factors and drug targets for thousands of phenotypes. Wellcome Open Res 2019;4:113. [Crossref] [PubMed]
- Verbanck M, Chen CY, Neale B, et al. Detection of widespread horizontal pleiotropy in causal relationships inferred from Mendelian randomization between complex traits and diseases. Nat Genet 2018;50:693-8. [Crossref] [PubMed]
- Hemani G, Zheng J, Elsworth B, et al. The MR-Base platform supports systematic causal inference across the human phenome. Elife 2018;7:e34408. [Crossref] [PubMed]
- Geller LT, Barzily-Rokni M, Danino T, et al. Potential role of intratumor bacteria in mediating tumor resistance to the chemotherapeutic drug gemcitabine. Science 2017;357:1156-60. [Crossref] [PubMed]
- Pushalkar S, Hundeyin M, Daley D, et al. The Pancreatic Cancer Microbiome Promotes Oncogenesis by Induction of Innate and Adaptive Immune Suppression. Cancer Discov 2018;8:403-16. [Crossref] [PubMed]
- Half E, Keren N, Reshef L, et al. Fecal microbiome signatures of pancreatic cancer patients. Sci Rep 2019;9:16801. [Crossref] [PubMed]
- Yang Q, Zhang J, Zhu Y. Potential Roles of the Gut Microbiota in Pancreatic Carcinogenesis and Therapeutics. Front Cell Infect Microbiol 2022;12:872019. [Crossref] [PubMed]
- Kandikattu HK, Venkateshaiah SU, Mishra A. Chronic Pancreatitis and the Development of Pancreatic Cancer. Endocr Metab Immune Disord Drug Targets 2020;20:1182-210. [Crossref] [PubMed]
- Zhou Q, Tao X, Xia S, et al. T Lymphocytes: A Promising Immunotherapeutic Target for Pancreatitis and Pancreatic Cancer? Front Oncol 2020;10:382. [Crossref] [PubMed]
- Thomas RM, Gharaibeh RZ, Gauthier J, et al. Intestinal microbiota enhances pancreatic carcinogenesis in preclinical models. Carcinogenesis 2018;39:1068-78. [Crossref] [PubMed]
- Sethi V, Kurtom S, Tarique M, et al. Gut Microbiota Promotes Tumor Growth in Mice by Modulating Immune Response. Gastroenterology 2018;155:33-37.e6. [Crossref] [PubMed]
- Lugea A, Waldron RT, Mareninova OA, et al. Human Pancreatic Acinar Cells: Proteomic Characterization, Physiologic Responses, and Organellar Disorders in ex Vivo Pancreatitis. Am J Pathol 2017;187:2726-43. [Crossref] [PubMed]
- Tsukamoto M, Imai K, Ishimoto T, et al. PD-L1 expression enhancement by infiltrating macrophage-derived tumor necrosis factor-α leads to poor pancreatic cancer prognosis. Cancer Sci 2019;110:310-20. [Crossref] [PubMed]
Cite this article as: Zhou C, Xu X. Causal relationship between gut microbiota and pancreatic cancer: a two-sample Mendelian randomisation study. Ann Pancreat Cancer 2024;7:1.